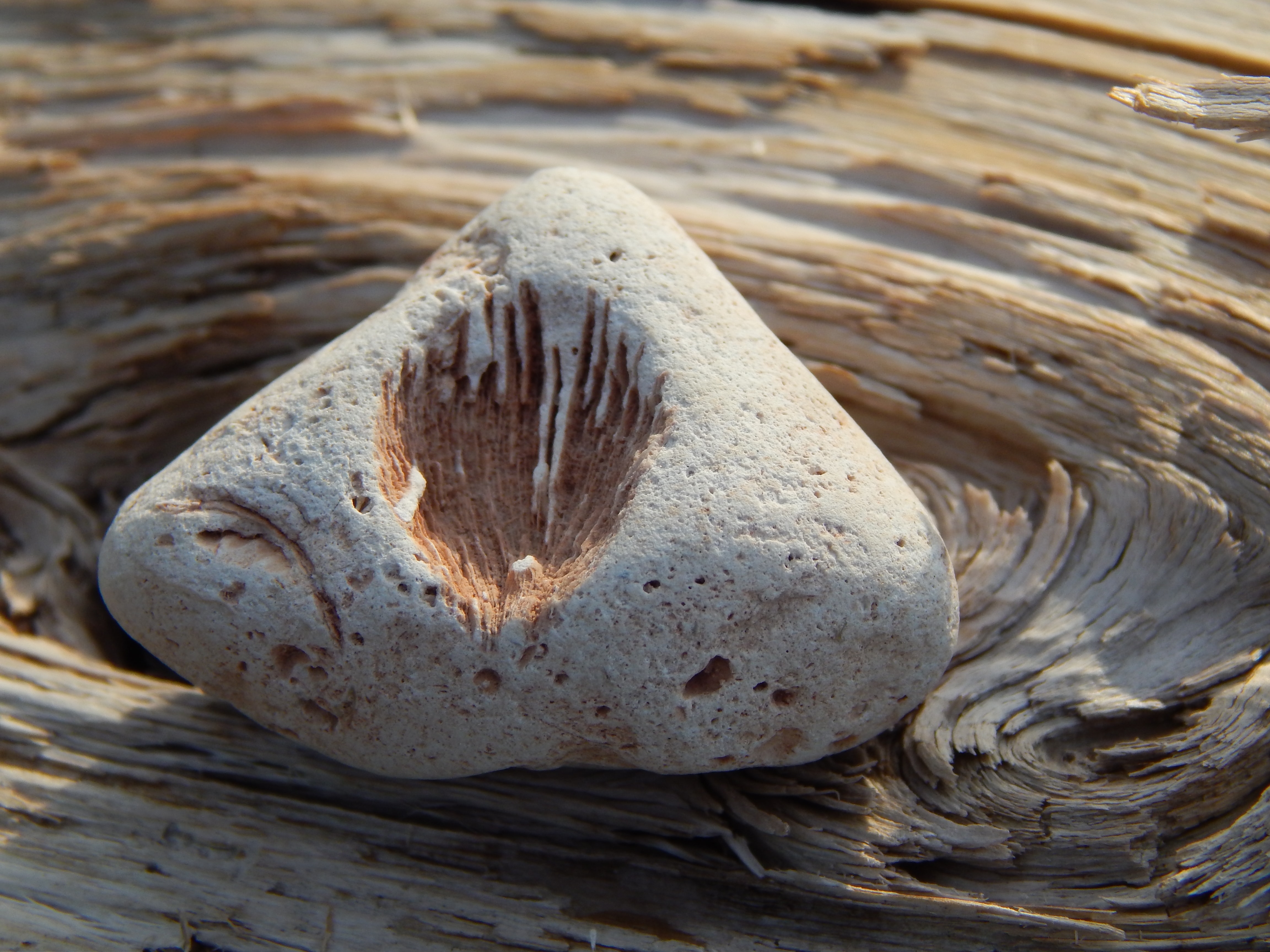
Almost two and half millions of years ago, the microscopic marine plankton species Globoconella puncticulata went extinct during a period of intense glacier formation across the Northern Hemisphere. Why did G. puncticulata go extinct when other ecologically similar species survived, including some to the present data? And what might its story have to teach us about the future of life under climate change today? Certainly the glaciers are retreating rather than growing across the Northern hemisphere right now, but perhaps there are more general insights to be gleaned from how species evolve (or not) in response to large environmental trends. Our project brings new mathematical modeling tools to bear on these questions, amplifying what we can learn about the directional evolution and responsiveness of species preserved deep in the fossil record.
Our team believes climate change poses an urgent and practical context for understanding the core concepts at the heart of the Science of Purpose initiative, including goal-directedness, agency, function, and directionality in living systems. As climate change accelerates, species across the planet are confronting shifting patterns of temperature and rainfall and experiencing more extreme weather events. How will life respond? Species rely on different cues from their environments for the timing of key life events, including flowering, hibernation, and growth, and in cases such as plants and pollinators the fate of one species is ecologically intertwined with others. Species are also differently able to move and adapt to new habitats as a function of their population sizes and how they reproduce and disperse. Some “living fossils,” for example, have survived relatively unchanged across millions of years but now occupy a habitat range that is only a small fraction of what they used to.
As recognized by the International Panel on Climate Change (IPCC), “the paleorecord can be used to derive fundamental rules by which organisms, ecosystems, environments and regions are typically most affected by climate change” (IPCC 2022). When scientists try to explain and predict how life will respond to new climates, much of their knowledge is based on ecological data collected over just a few decades. One use for going deeper in time to study the fossil record, then, is to extend our historical baseline of knowledge. Fossils, for example, let scientists calibrate estimates of viable species habitats against time periods where human influence on the landscape was less or absent entirely. Fossil evidence also expands our knowledge of what is possible when life confronts extreme environmental conditions.
In particular, our project aims to unlock new questions and insights by applying a new mathematical modeling framework to existing fossil data and to inform the design of future empirical studies. Marine invertebrate species such as G. puncticulata offer particularly exciting opportunities because they are abundant and widespread, making them much easier to find and collect compared to Tyrannosaurs Rex (although they’re admittedly not as cool to look at individually). In addition, it's possible to study fossilized marine invertebrates at the same rough location over tens of thousands of years by drilling into the ocean sea bed and collecting core samples. New computational approaches are also helping automate the identification of fossil species in these core samples and the measurement of traits such as the shell size and shape. Other innovative models are exploring the biophysics of shells to connect these basic morphological traits to functions.
The statistical methods paleontologists currently use, however, are not well-suited to integrative research that connects environmental changes to a multdimensional picture of how species are evolving. Cutting-edge work by Gene Hunt in the 2000s showed how to distinguish between different “modes” of evolutionary change for single traits measured on specimens from a fossil lineage. Reaching back to the debate on punctuated equilibrium, Hunt focused in particular on whether a lineage’s trait exhibited a pattern of random fluctuations around a constant mean, undirected random change, or random change showing a directional tendency. In more technical terms, biologists refer to these patterns as stasis, a random walk, and a directional random walk, respectively.
By matching empirical patterns to different mathematical models for trait evolution, fossils provide uniquely valuable insights into how the day-to-day outcomes of organisms struggling to survive and reproduce add up to grand patterns on the scale of millions of years. Charles Darwin, who pioneered the idea of natural selection in the 1800s, thought species evolved by gradually accumulating many small beneficial changes. More recently, though, the evolutionary biologists Niles Eldredge and Stephen J. Gould hypothesized in 1973 that populations accumulate change primarily when they speciate, i.e. split into new species, and that otherwise their traits remain constant. They called this hypothesis “punctuated equilibrium” to capture the idea that change accumulates rapidly only during relatively brief periods of speciation that across happen much longer spans of constancy. The resulting debate among supporters of Darwin’s versus Eldredge and Gould’s hypotheses has been extraordinarily fruitful, motivating the collection of new fossil data and novel statistical models to analyze them.
However, Hunt and other scientists also recognized that there are multiple evolutionary processes that can cause the same overall pattern. A trait might exhibit stasis, for example, because it’s constrained by a lack of underlying genetic variation, by another trait, or by physical forces arising during development. Alternatively, a trait might exhibit stasis because it is close to the optimum fitness under natural selection. Similarly, a random walk might reflect simple chance mutations accumulating over time, or it could represent a population evolving toward a fitness optimum that itself is fluctuating randomly over time. In short, paleontologists have recognized there is no simple way to go from the best-fit pattern for how a single trait is changing to conclusions about the causes responsible for that pattern.
Being able to detect and quantify multivariate relationships among traits and environmental variables would therefore enable novel connections between biological theory and data and provide a more robust foundation for documenting patterns of directional change in evolutionary history. Dynamic Linear Models (DLMs) can fill this gap by providing an accessible modeling framework for paleobiologists with a wide range of advantages for model estimation, validation, and analysis. While DLMs are an established, widely used approach to statistical modeling of time series, they have been overlooked for fossil lineages. In addition to making multivariate models possible for a much larger number of lineages, DLMs can also expand our ability to detect important processes even for traits that currently lack clear ecological or evolutionary significance, as is common for marine invertebrate lineages. Establishing procedures for testing latent processes, i.e. in the absence of known external drivers, will enable paleobiologists to make the most of existing datasets and help target future empirical research.
Introducing more complex biological models into our analytical toolkit, though, also raises novel challenges for biologists at the intersection of statistical methodology and philosophy of science. Many biologists seek to falsify hypotheses about evolutionary patterns and processes, for example, but contemporary model selection practices in evolutionary biology commonly lack robust tools and practices for quantifying and controlling error probabilities. In practice, the ways biologists understand evidence are entangled with limitations in the methods they have available. Nonetheless, conclusions about whether a fossil lineage trait exhibits stasis or gradual directed change, for example, should be objective in the sense of not varying from one investigator to the next due to differences in the background assumptions. Nonetheless, recent studies have shown that scientists using the same data and candidate statistical models can arrive at conflicting empirical conclusions because they disagree about whether the models are adequate to the complexity of the biological phenomena at hand. Our project therefore will also use the DLM framework to investigate how empirical conclusions about directionality, for example, are sensitive to differences in investigator background assumptions, and how innovative approaches to quantifying evidence in statistical model selection can help address these concerns.